3 Reasons to Switch from Manual to Automated Financial Data Analysis for Fraud Investigation (with Solution)
Bernie Madoff ran a Ponzi scheme that may have lasted nearly 40 years. Enron hid hundreds of billions of dollars in debt for over four years. The perpetrators of these fraudulent schemes had something in common — they almost avoided being caught.
Despite dealing with sophisticated investors, both Madoff and Enron hid their fraudulent activities by generating a mountain of false data. Madoff provided six pages of false trading accounts in response to an early investigation in 1999. The U.S. Securities and Exchange Commission never picked through the trading accounts manually to determine whether the accounts or trades were genuine.
Enron also generated boxes and boxes of documents to obscure its activities. The U.S. Federal Bureau of Investigation took six years to analyze Enron’s books to identify billions of dollars in fraudulent transactions.
These cases expose the major issues faced when manually analyzing financial data for investigation. Manual analysis is slow, prone to errors, and can obscure the patterns needed to identify fraud.
After these failures in the late 1990s and early 2000s, law enforcement agencies like the IRS and the FBI increased their reliance on digital fraud analysis tools. Digital analysis has revolutionized financial investigations. The tools that were available only to law enforcement agencies are now available to private firms as well.
The same artificial intelligence that your phone uses to recognize your fingerprint can also be used to recognize and import financial transactions. This can increase the efficiency and accuracy of financial analysis, leaving you more time to identify fraudulent patterns.
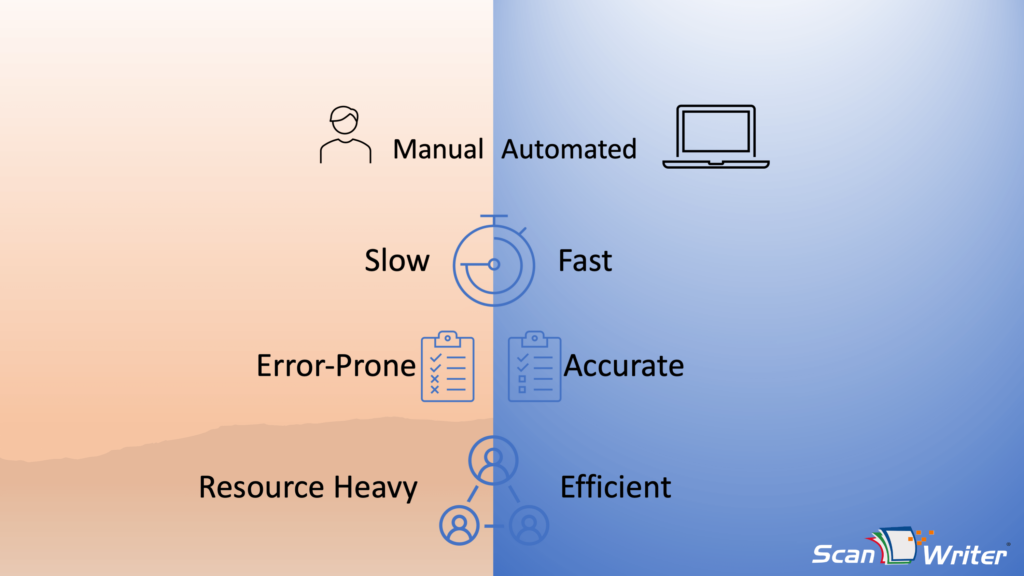
Shortcomings of Manual Analysis of Financial Data
Not every case involves hundreds of billions of dollars in fraud. A tax assessor might need to analyze whether a local business has been hiding assets or income. A police detective might need to analyze the firm’s books to determine if an employee has embezzled funds. A city auditor might suspect that vendors and contractors have provided misleading records about projects performed for the city.
In these cases, a fraud analysis might not take six years of investigation like the Enron case. But it may still involve years of records with hundreds or even thousands of transactions every month.
#1 Manual Financial Analysis is Time Consuming
Almost every case, even a simple case, requires time to prepare and integrate data. This could involve days, weeks, or even months to place the data in a condition for analysis.
More importantly, you cannot rely on the company’s books or accounting ledgers. If you suspect fraud, the fraud might be baked into the electronic ledgers. Instead, you must rely on third-party records including:
- Bank statements
- Brokerage statements
- Checks
- Deposit slips
- Receipts
- Invoices
- Credit card statements
- Payroll records
- Loan statements
After you gather these records, you must input them into spreadsheets, normalize them, clean them up, and integrate them into a common spreadsheet for analysis.
The time spent just to assemble the data for analysis can delay an investigation. An investigation can cost more than expected in such a scenario. Manual data entry and analysis require human resources like data entry clerks and quality assurance managers. Finding and employing qualified workers will take time and cost money.
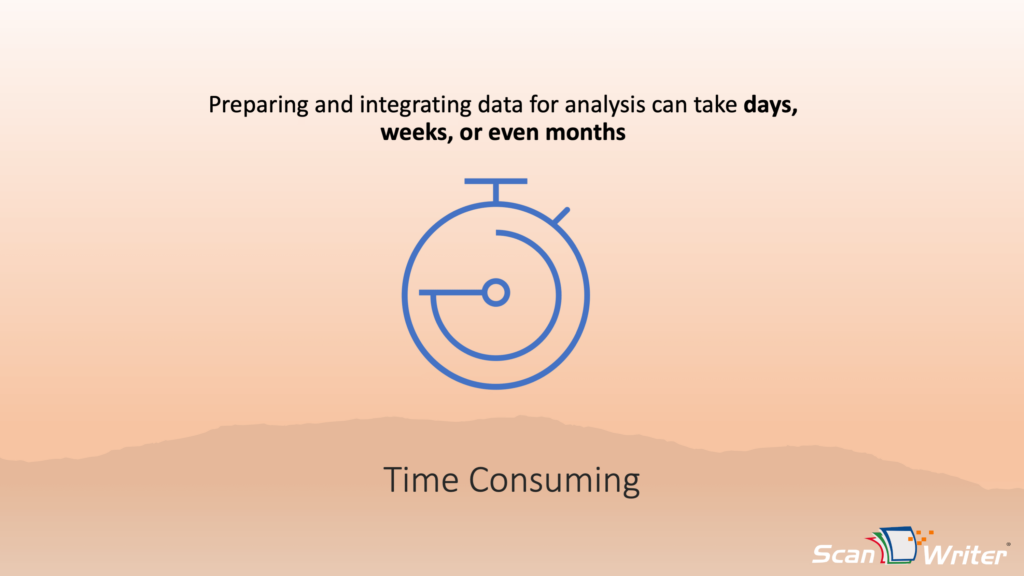
#2 Manual Financial Analysis Could be Error-Prone
While converting the data from paper to electronic, errors will be introduced. This is not speculation. You will have errors in the transcription. You will need to find the errors. And you will need to verify that the errors have been rectified.
But even more important are the errors you do not know exist until you are deep into your analysis. Missing data and duplicate data might fly under the radar until you verify your analysis manually. Then you might find that you have wasted days, or even weeks, working with error-filled data.
Worse yet, you might not find the errors in the data and produce an erroneous analysis. A false accusation of fraud can lower your reputation as a fraud analyst. It can also bring legal actions against you for shoddy work.
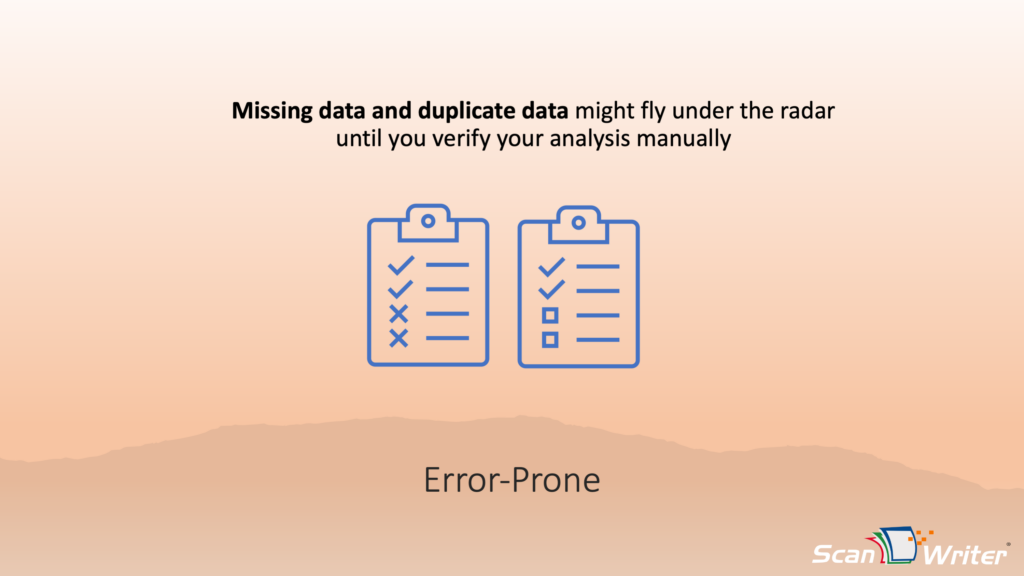
#3 Manual Financial Analysis Could be Resource Heavy
In addition to costing more than it should, manual analysis forces you to allocate resources to data preparation instead of data analysis. While a solid analysis requires good data, spending fewer resources on data preparation will allow you to allocate more resources to data analysis. This helps you allocate your municipal resources to meaningful analysis rather than wasting them on data preparation.
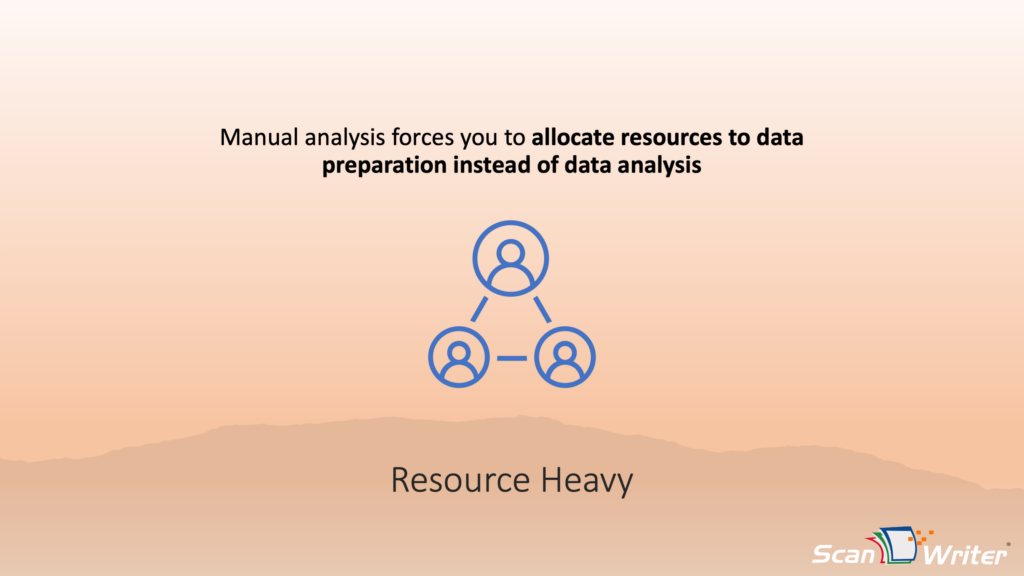
An Accurate and Efficient Alternative | Financial Investigation Software
ScanWriter – a financial investigation software – extracts data from multiple types of documents. These data are used to create a transactional database that integrates all the records captured. That is, instead of just recognizing the text like an OCR software package might, it recognizes the transactions and imports them into a spreadsheet for analysis.
ScanWriter provides several advantages over manual data preparation and analysis including:
#1 Financial Analysis Automation
By using ScanWriter, you will not need to develop financial models for analyzing the data. Instead, ScanWriter offers various financial models for a financial investigation.
When you review financial transactions for signs of fraud, you probably use spreadsheet functions like pivot tables, sort, and filter. These features can show you where the money comes and goes. They can also reveal transaction patterns. But they are not customized to accounting or fraud investigations
ScanWriter offers additional features that supplement or even replace the spreadsheet tools you use for fraud analysis. The software has built-in features such as data normalization, automatic accuracy check, and functionality to export your results to Excel or Power BI in one click.
This last feature allows you to take advantage of powerful models built into ScanWriter specifically for financial fraud analysis. One of these models, called “Flow of Funds,” gives you a visual representation of where the money comes and goes. It allows users to identify where the money comes and goes to support their investigation for a case.
Importantly, these models are user-friendly. Anyone can use them, even without a background in data analytics or computer science.
This puts the data where you need it for your analysis rather than hunting around for the relevant data or spending time manually inputting the data into the desired model.
#2 Accuracy of Financial Data
Accuracy is a critical component of fraud analysis. You must correctly identify fraudulent transactions and patterns of fraudulent activity. This analysis must stand up to counter-analysis and cross-examination by the person or business accused of fraud.
You must also avoid making false accusations of fraud. Bad data preparation can introduce errors that could mimic fraud. By improving the accuracy of your data preparation, you can reduce the risk of false positives as you analyze the financial data.
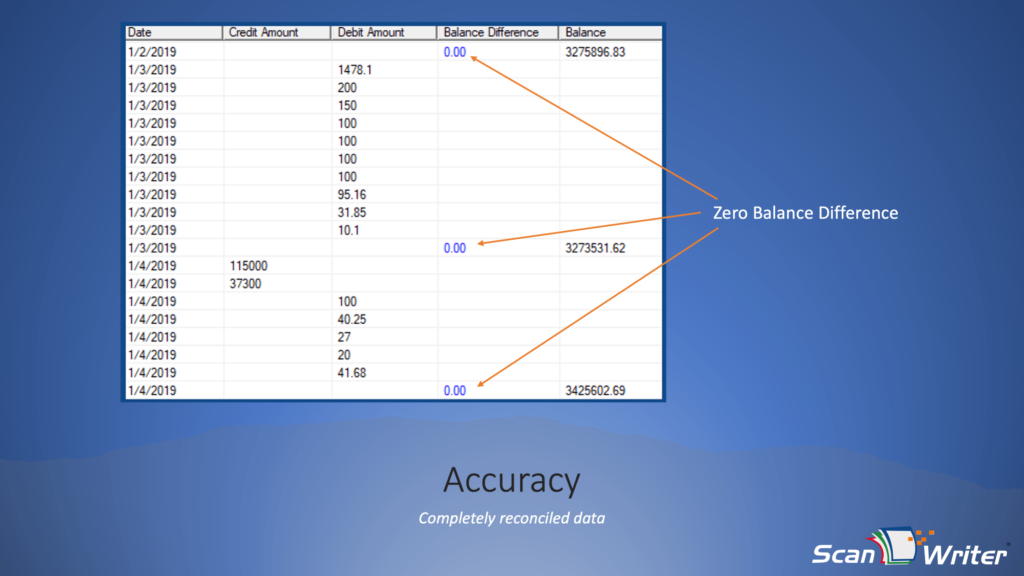
#3 Audit Trail (Verification of Financial Data with Original Source)
ScanWriter allows verification with the source document in one click. The working data in the spreadsheet will directly correlate to the optically scanned data in the original document. This allows you or your staff to verify that questionable transactions were captured accurately.
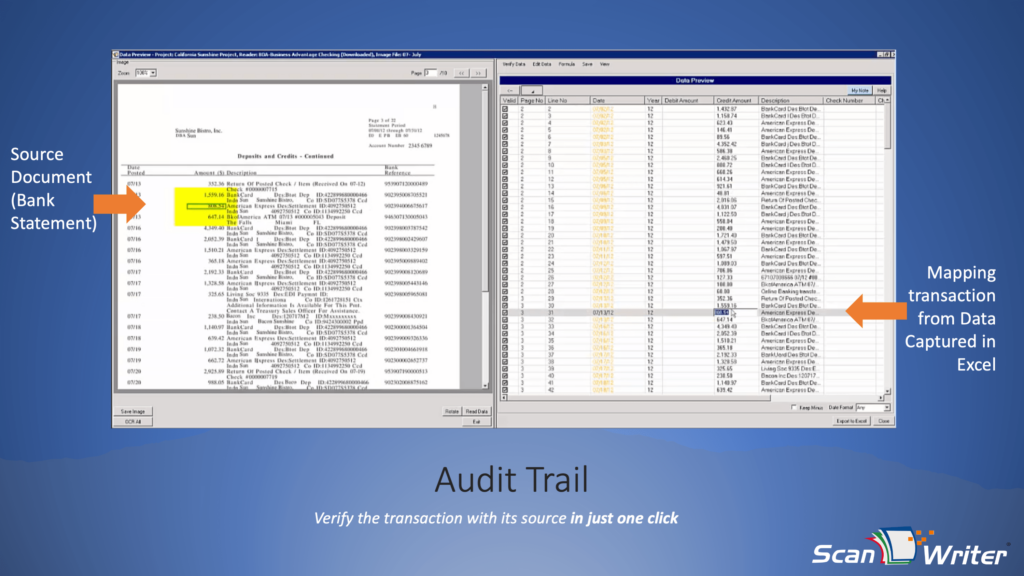
#4 No False Positives (Eliminate Dupicate Data)
ScanWriter also identifies duplicate data automatically. One signifier of potentially fraudulent activity is a pattern of repeated transactions. By automatically eliminating duplicate data, you eliminate this source of false positives.
#5 Assistance with your Financial Investigation
By improving accuracy and speed through automation, ScanWriter helps you produce better analysis. Some ways ScanWriter assists your fraud investigations include:
A. Speed: By automating the data preparation process, you can start your financial analysis faster. Moreover, your analysis will proceed more quickly because ScanWriter can provide various financial models for the data.
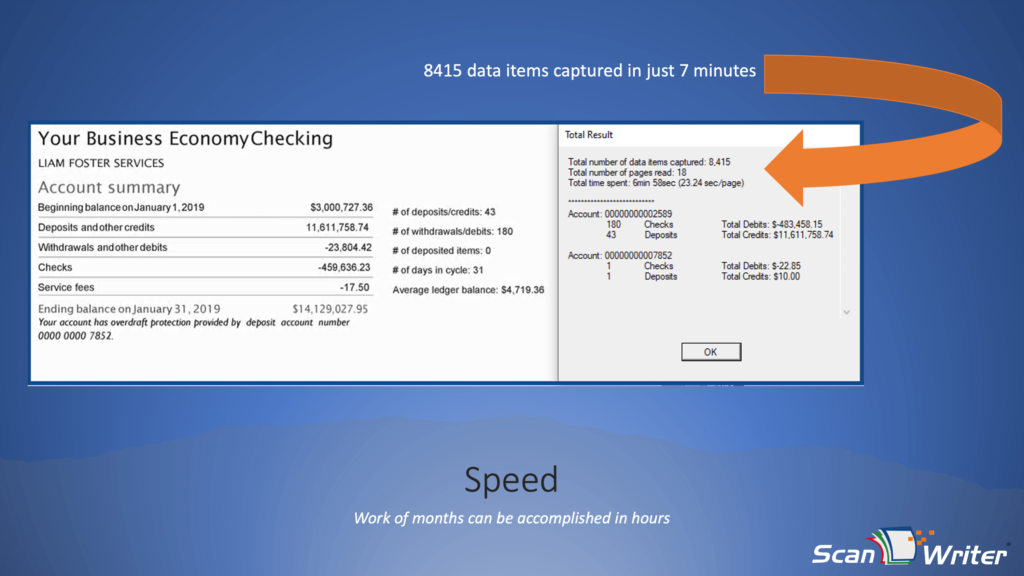
B. Identify unmatched transfers: Rather than combing through the records entry by entry, ScanWriter can identify unmatched transfers that often signify fraudulent activity.
C. Identify undisclosed accounts: By cross-matching the data from various accounts, ScanWriter can identify missing or undisclosed accounts involved in the recorded transactions.
#6 Security of the Financial Data
One concern during a fraud investigation is the integrity of the data. Someone accused of fraud could raise questions about your handling of the data to impugn your analysis.
ScanWriter can be used as desktop software. This preserves the integrity of the data and deprives fraudsters of this counterargument.
#7 Seamless Integrations with other Financial Softwares
ScanWriter integrates seamlessly with Excel, and Microsoft Power BI to facilitate analysis as shown below.
Integration with Excel
Integration with Microsoft Power BI
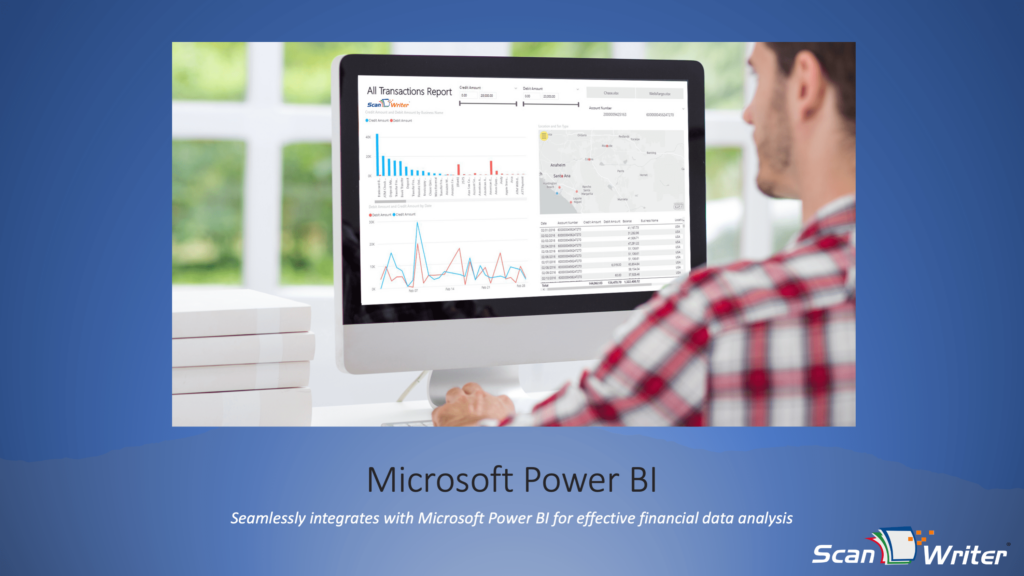
#8 Capacity of Configuring 15000+ Financial Institutions
ScanWriter is configured to interface with 15000+ financial institutions. If your analysis involves records from an institution that’s not in ScanWriter’s database, a new financial institution or bank can be added to the software suite in 24-48 hours.
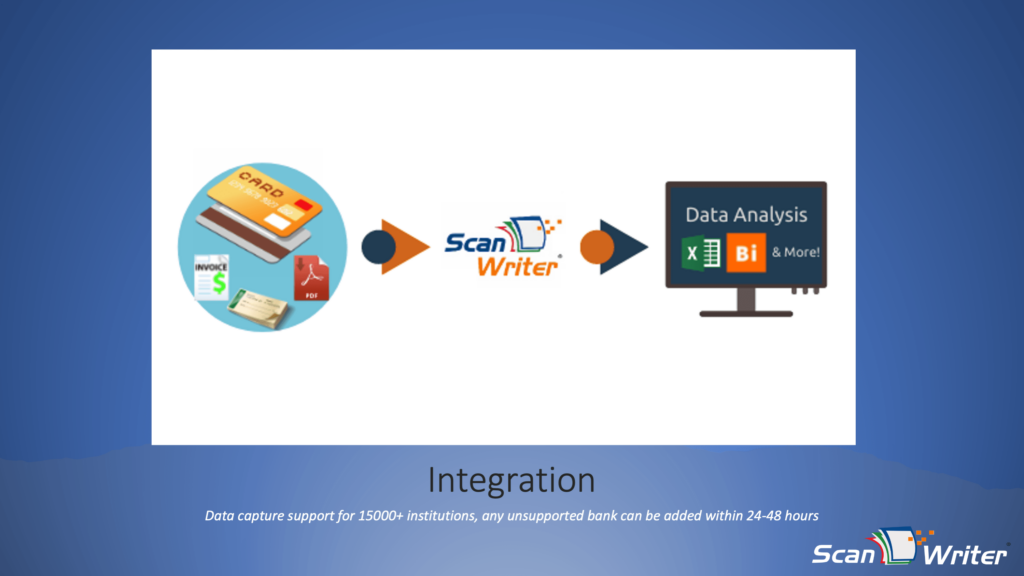
#9 Multiple Language Support
ScanWriter can gather information from documents in 21 languages including English, Spanish, and other major European, Asian, and Middle Eastern languages.
Paradigm Shift to Automated Financial Data Analysis for Fraud Investigations
The future of fraud analysis will come from automation. Automated financial data analysis will not only make you faster and more accurate but free you from the mundane tasks of data preparation so you can focus on data analysis.
To learn more about how ScanWriter can improve your fraud investigations, request a ScanWriter Demo.